Disparate impacts on online information access during the Covid-19 pandemic
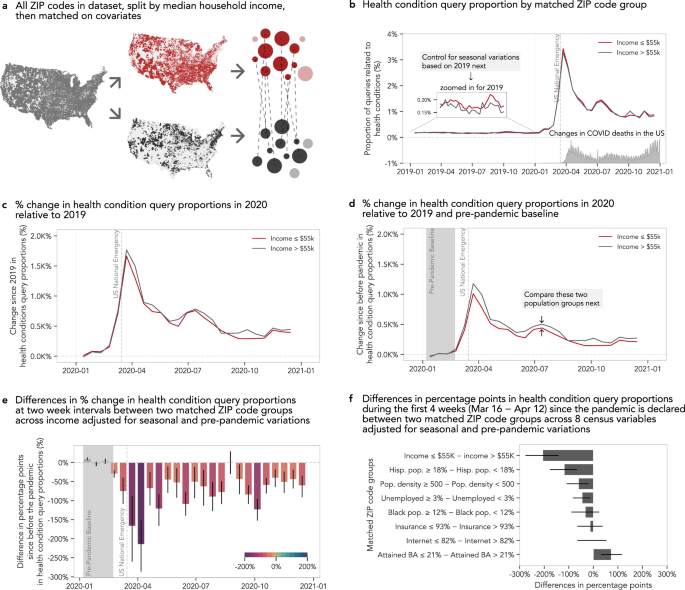
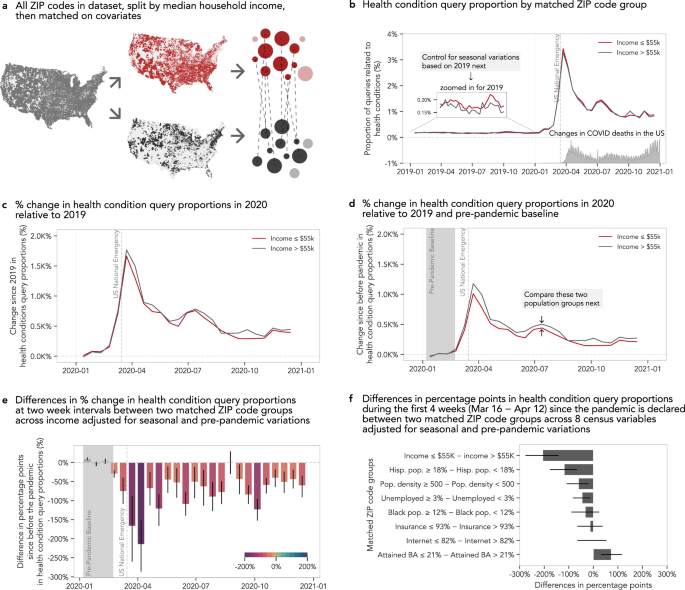
Data set and study population
Our source dataset consists of a random sample of 57 billion de-identified search interactions in the United States from the years 2019 and 2020 from Microsoft’s Bing search engine. Each search interaction includes the search query string, URLs of all subsequent clicks from the search result page, timestamp, and ZIP code. We excluded search interactions from ZIP codes with less than 100 queries per month so as to preserve anonymity. Our search dataset intentionally includes both desktop and mobile Bing search interactions in order to capture both search query sources. Although the quality of access, especially through different device types or device specifications, has been highlighted as another important factor in recent digital divide research13, analysis on the differential search behaviors across device types is outside the study’s scope. All data were de-identified, aggregated to ZIP code levels or higher, and stored in a way to preserve the privacy of the users and in accordance to Bing’s Privacy Policy.
While many Americans use other search engines such as Google, Bing’s query-based market share is estimated to be ~26.7% according to Comscore data93. We focused on query-based metrics for estimating search market share because it captures end-users’ interaction with the search engine, including queries that may not have resulted in site visits. Click share, on the other hand, captures only search-driven traffic to a subset of websites that are instrumented with custom code. To understand the validity of relying solely on Bing search data, we compared Bing and Google queries for matched categories longitudinally and found that the search trends are highly correlated (Pearson r = 0.86 to r = 0.98, Supplementary Fig. 2). Our search ZIP code data is provided by a proprietary location inference engine, with added accuracy improvements to standard reverse IP lookup databases from contextual and historical information, but such estimation is still an approximation. Our study also assumes that the demographics of the search users in a ZIP code reflect the demographics of the population within a ZIP code. However, search users generally trend towards more white, richer, and older population. It is difficult to accurately characterize the population base without third-party services such as Comscore data93, which may have its own limitations and biases. Our analysis of a proportion of user demographics using such data confirms that Bing data tracks the US population reasonably well.
The study (protocol ID 632) was reviewed by the Microsoft Research Institutional Review Board (OHRP IORG #0008066, IRB #IRB00009672) prior to the research activities. Microsoft Research is an industry-based research institution with a United States Department of Health, Human Services (HHS) federally registered IRB. In addition to following federal ethical research guidelines, Microsoft Research IRB takes an anthropological stance in looking at the impacts of research and looks beyond the risks to human subjects, according to IRB regulations, but also risks to human society94. The authors and the Microsoft Research IRB recognize the sensitive nature of the use of data collected from Microsoft users for research purposes. Our study followed the privacy and security regulations governed by Microsoft’s privacy statement as well as the federal ethical guidelines set forth by the HHS. All search data have been de-identified and aggregated prior to receipt by our study team such that no identifiable information was processed or analyzed. Via a standard ethical review process prior to the study, Microsoft Research IRB formally approved our study as “Not Human Subjects Research” to indicate that the activities do constitute research, but where the definitions of “human subject” and “identifiable private information” do not apply (as defined by 45§46.102(e)). Microsoft Research IRB certifies that our Human Subjects Review process follows the applicable regulations set forth by the Department of Health and Human Services: Title 45, Part 46 of the Code of Federal Regulations (45 CFR 46) (the Common Rule), and our Ethics Program promotes the principles of the Belmont Report in our research institution. In addition to the ethics review, our study obtained approvals from Microsoft’s privacy, security, and legal review officers prior to obtaining and analyzing the data.
ZIP code level data
One of our goals is to characterize the role of socioeconomic and environmental factors on digital engagement outcomes. Unfortunately, data that combines individual-level search interactions with each individual’s socioeconomic and environmental characteristics at the US national scale does not exist, is difficult to capture, and invites privacy concerns. Instead, we use ZIP codes as our geographic unit of analysis. ZIP code level analysis can be limited because it cannot describe each individual living in those ZIP codes. However, ZIP code level analysis can scale to nontrivial population sizes and has been repeatedly recognized and leveraged in population-scale and local/neighborhood-level research20,95,96,97,98,99,100. ZIP code level analysis also enables accounting for well-known issues associated with residential segregation and socioeconomic disparities12,101. We leveraged the available ZIP code level American Community Survey estimates using the Census Reporter API102 in order to characterize the ZIP codes in our dataset.
Census variables and search categories
We chose a set of census variables to delineate ZIP code groups as well as search categories to examine digital behaviors. Supplementary Fig. 1 illustrates our full choice of census variables and search categories.
The SDoH has been widely used as a holistic framework to describe a broad range of socioeconomic and environmental factors that determine one’s health, well-being, and quality of life. In recent years, the SDoH has also been referenced in relation to digital divide; digital literacy and internet access are referred to as super determinants of health as they relate to all social determinants of health86. Just as Helsper57 theorized the corresponding digital and offline fields, looking at variables from both offline and digital aspects of the social determinants of health are critical in understanding digital disparities. Because of the multidimensional nature of socioeconomic status and its association with health and well-being outcomes, it is important to include relevant socioeconomic factors91. Therefore, our choice of census variables and search categories are largely influenced by the SDoH framework defined by the US Department of Health62.
We considered multiple socioeconomic factors including race, income, unemployment, insurance coverage, internet access, educational attainment level, population density, age, gender, Gini index, homeownership status, citizenship status, public transportation access, food stamp, and public assistance. We did not include some of the factors when they were highly similar to already included factors (e.g., % below poverty level is correlated to median household income, Pearson r = − 0.624). In the end, we included eight census variables that represent all five categories of SDoH to cover a broad range of socioeconomic and environmental factors.
Under Healthcare Access and Quality, we included the percentage of the population with health insurance coverage (Table B27001). Under Education Access and Quality, we included the percentage of the population that attained a Bachelor’s degree or higher (Table B15002). Under Social and Community Context, we included the percentage of the population of Hispanic origin (Table B03003) and the percentage of the population with Black or African American alone (Table B02001). Under Economic Stability, we included the median household income (Table B19013) and the percentage of the civilian labor force that is unemployed (Table B23025). Under Neighborhood and Built Environment, we included the percentage of the population with a broadband or dial-up internet subscription (Table B28003) and the population density. We computed per ZIP code population density by joining area measurements from ZIP Code Tabulation Areas Gazetteer Files103 and total population (Table B01003). We joined the search interaction data with the above SDoH factors on ZIP codes and excluded ZIP codes that did not have either search interactions or census data. The resulting 55 billion search interactions covered web search traffic from 25,150 ZIP codes in the US, and these ZIP codes represent 97.2% of the total US population. Supplementary Table 1 provides per-ZIP code summary statistics of our dataset.
Our choice of search categories was largely informed by our prior work9. We chose three determinants—Healthcare Access and Quality, Education Access and Quality, and Economic Stability—from which to draw our search categories. We excluded two determinants that were generally more difficult to capture with simple query string matches because they tend to be more contextual (e.g., location, social) than can be expressed as query strings for information-seeking. Under the three SDoH factors, we chose seven search categories that not only appeared more frequently than others in our dataset but also were relevant topics during the pandemic. Supplementary Table 4 enumerates the categories we examined with example query strings, URLs, and regular expressions.
Examining individual search keywords or subcategories has been pursued by others within and outside the scope of the pandemic. In our study, the use of broad categories spanning health, economics, education, and food is intended to capture a holistic view of the pandemic across many different needs61. Accordingly, we do not make any claims about subcomponents within a category because studying these subcomponents is out of scope of this work.
Certainly, there exist search keywords that are more popularized by the current pandemic, such as “coronavirus” or “covid”, that also belong in the health information category. However, these keywords are not unique to the current pandemic and have existed before. As infrequent searches for “coronavirus” might seem in 2019, in our data, the query frequency of “coronavirus” in 2019 was similar to that of “mers” and certainly not zero (Supplementary Fig. 3). In fact, many categories of interests exhibited changes during the pandemic9,19, not just some that are highly relevant to the pandemic. For example, Suh et al.9 has demonstrated that many of the ordinary search topics, such as “toilet paper”, “online games with friends”, or “wedding” were significantly changed during the pandemic.
Disproportional change in digital engagement during the pandemic
Our goal is to quantify the disproportional change in digital engagement during the pandemic experienced by different subpopulations. Our study conducts several data processing steps and analysis methods to arrive at our findings: (1) we quantify digital engagement by computing relative query proportions for various search categories, (2) we quantify intensification or attenuation of digital engagement by computing changes in digital engagement between before and during the pandemic, and (3) we compare the changes in digital engagement across ZIP code groups.
Digital engagement trends
We leverage interactions with search engines to obtain signals about digital engagements where everyday needs are expressed or fulfilled through a digital medium, in our case Bing9. In our study, we characterize digital engagement through modeling users’ search interests as expressions of underlying human needs9, building upon prior work that uses search interactions to model interests that are either expressed explicitly through search queries or implicitly through clicks on results displayed on the search engine result page16,17,18. To gain a nuanced understanding of these search interactions, we categorize each search interaction into topics ranging from health access, economic stability, and education access. We match each search interaction to a corresponding category through simple detectors based on regular expressions and basic propositional logic (Supplementary Table 4). Each category could have multiple regular expressions applied to either the query string, the clicked URL, or both. Then, we count matching search interactions for a given category. Our query string detectors operate only on English-language keywords such that any cross-cultural or cross-language analysis is out of scope of this work, but some of our detectors include looking at the click results regardless of the query.
To capture the level of search interest in these categories in relation to all other categories of interest, we compute the proportion of total search queries that belongs to a specific category. For example, we compute the proportion of total search queries that contain health condition keywords such as cancer, diabetes, or coronavirus to quantify the level of interest in engaging in health information-seeking behaviors in relation to all other digital engagement behaviors. In another case, we examine search queries that result in subsequent clicks to state unemployment benefit sites to quantify the level of interest in unemployment benefits.
In addition, the focus on the level of interest through query proportions rather than query frequencies is helpful in our analysis. First, it helps with accounting for the baseline differences in search access between two populations. Second, this focus on relative measures of search query frequency helps adjust for changes in query volume over time, which is a common practice in Information Retrieval and web search log analysis104,105. Supplementary Fig. 4–11 illustrate the temporal variations in relative query frequencies (left) and in relative query proportions (right) in each query category for each of the two matched groups across all SDoH factors. Adjusting for the baseline differences in search access allows us to remove the existing access differences between the two groups, and the temporal trends of the query proportions between the two groups become much closer.
Longitudinal before and during pandemic change in digital engagement
To capture longitudinal changes in search behaviors that are most likely attributable to the pandemic, we use a difference-in-differences (DiD) method64 to apply several corrections. DiD is often used in econometrics and public health research as a quanti-experimental research method to study causal relationships where a randomized control trial (RCT) is infeasible106. Using DiD design with the pandemic as the treatment cannot lead to any causal claims because there is no control group or a counterfactual (i.e., everyone is exposed to the pandemic). In our study, we leverage DiD method to quantify the intensification or attenuation in search behaviors by removing seasonal variations and normalizing on pre-pandemic baselines.
After we categorize each search interaction with our categories of interest, we count and aggregate them per time window (i.e., 2-week or 4-week intervals in our analysis) and per ZIP code (Fig. 1a). We compute the proportion of the total query volume represented by each category for these time windows to quantify the level of search interests in that category while removing undesired variations in the query volume over time (Fig. 1b). We denote the digital engagement at time t in category c as the fraction of the total number of queries at time t: E(t, c) = N(t, c)/N(t). From this, we control for yearly seasonal variations by subtracting the digital engagements of 2019 from that of 2020: E(t2020, c) − E(t2019, c). People tend to behave differently on weekends, and we observed a 7-day periodicity in our data, sometimes known as the “weekend effect”107. Therefore, when comparing two years, it is important to account for the weekend effect. In order to highlight the actual differences that are not explained by weekend mismatches across years, we aligned the day of the week between both years (i.e., Monday, 6 January 2020 is aligned to Monday, 7 January 2019). In addition, we ensured that our comparison analysis included all seven days of the week (i.e., look at means across one or multiples of a full week) (Fig. 1c).
Finally, to compute the change in digital engagement during the pandemic since the time at which the US national emergency was declared on 16 March 2020, we subtract the query proportions between 6 January 2020 and 23 February 2020, a period we defined as the “pre-pandemic baseline” (Fig. 1d). Even though the national emergency was declared three weeks later, we use 23 February 2020 as the cutoff because individual states declared a state of emergency at different times between February 29 and March 15 of 2020 and to avoid partial weeks in our analysis. Our estimate of the relative change in digital engagement in category c between before and during the pandemic is defined as:
$$C({t}_{{{{{{{{rm{before}}}}}}}}};{t}_{{{{{{{{rm{during}}}}}}}}},c)=left[Eleft({t}_{{{{{{{{rm{during}}}}}}}}}^{2020},cright)-Eleft({t}_{{{{{{{{rm{during}}}}}}}}}^{2019},cright)right]\ -left[Eleft({t}_{{{{{{{{rm{before}}}}}}}}}^{2020},cright)-Eleft({t}_{{{{{{{{rm{before}}}}}}}}}^{2019},cright)right]$$
(1)
Or the relative percentage change in digital engagement Cperc is expressed as:
$$ {C}_{{{{{{{{rm{perc}}}}}}}}}({t}_{{{{{{{{rm{before}}}}}}}}};{t}_{{{{{{{{rm{during}}}}}}}}},c)\ =frac{left[Eleft({t}_{{{{{{{{rm{during}}}}}}}}}^{2020},cright)-Eleft({t}_{{{{{{{{rm{during}}}}}}}}}^{2019},cright)right]-left[Eleft({t}_{{{{{{{{rm{before}}}}}}}}}^{2020},cright)-Eleft({t}_{{{{{{{{rm{before}}}}}}}}}^{2019},cright)right]}{left[Eleft({t}_{{{{{{{{rm{before}}}}}}}}}^{2020},cright)-Eleft({t}_{{{{{{{{rm{before}}}}}}}}}^{2019},cright)right]}times 100$$
(2)
We acknowledge that there may exist a ZIP code with zero or very little search interactions for a given category, especially before the pandemic and in 2019. For example, “stimulus check” may only be relevant during the pandemic for certain ZIP codes. We cannot exclude these ZIP codes because we want a good representation and distribution of ZIP codes in our analysis. If a ZIP code makes only a handful of search queries on various health conditions, for example, but the number of queries increases dramatically due to concerns surrounding comorbidities and health complications, that is precisely the signal we hope to capture and observe across ZIP code groups. We mitigate this potential challenge of zero or near-zero baseline issues in several ways. (1) Our regular expressions are inclusive of potential variations in expressing the categories, including expressions that are likely to occur before the pandemic and in 2019. (2) We aggregate search interactions in two or 4-week windows, which consequently reduces the likelihood of having no or very little search interaction before the pandemic. (3) We also aggregate across thousands of ZIP codes that belong to a specific group (e.g., a group of ZIP codes with median household income greater than $55,224), where the likelihood of having no or very little search interaction before the pandemic for each group is 0%. (4) Instead of computing per-ZIP code DiD, we compute per-group DiD. In other words, we perform a within-group summation before taking the difference, which allows us to characterize the change in digital engagement for a typical ZIP code in the group.
Comparisons across ZIP code groups
Finally, we aggregate these changes in digital engagements across two comparison ZIP code groups for each SDoH factor, for example, to compare the average change in digital engagement of low-income ZIP codes with the average change of the high-income ZIP codes (Fig. 1d). Thus, we operationalize the disproportional change in digital engagement during the pandemic by quantifying the differences in the changes in search behaviors for a single search category between two ZIP code groups delineated by a single SDoH factor (Fig. 1). In our analysis, we report the change in digital engagement as the percentages of the pre-pandemic baseline, Cperc, where 0% denotes no change. We report the disparities in the changes in digital engagement between two comparison ZIP code groups as the percentage point difference where 0 denotes no difference (Fig. 1e, f). We formalize disparities in the changes in digital engagement in category c during the pandemic between high-risk ZIP code group ghigh and low-risk ZIP code group glow as:
$${D}_{{{{{{{{rm{perc}}}}}}}}}({t}_{{{{{{{{rm{before}}}}}}}}};{t}_{{{{{{{{rm{during}}}}}}}}},{g}_{{{{{{{{rm{low}}}}}}}}};{g}_{{{{{{{{rm{high}}}}}}}}},c)={C}_{{{{{{{{rm{perc}}}}}}}}}^{{g}_{{{{{{{{rm{high}}}}}}}}}}({t}_{{{{{{{{rm{before}}}}}}}}};{t}_{{{{{{{{rm{during}}}}}}}}},c)\ -,{C}_{{{{{{{{rm{perc}}}}}}}}}^{{g}_{{{{{{{{rm{low}}}}}}}}}}({t}_{{{{{{{{rm{before}}}}}}}}};{t}_{{{{{{{{rm{during}}}}}}}}},c)$$
(3)
To obtain non-parametric 95% confidence intervals, we conducted bootstrapping with replacement at 500 iterations during this aggregation step. These confidence intervals are computed when estimating the effect size (i.e., the difference between matched groups) and are visualized with figures demonstrating the difference between groups. All errors bars in figures indicate this 95% bootstrapped confidence interval (N = 500). Supplementary Figs. 13–26 illustrate percent changes in each query category for each of two matched groups and their differences in percentage points across all SDoH factors.
Matched comparison groups
Our goal is to quantitatively estimate the independent association between one socioeconomic factor and the changes in digital engagement while controlling for other factors during a global crisis such as the COVID-19 pandemic. Specifically, we are interested in eight SDoH factors: (1) median household incomes, (2) % unemployed, (3) % with health insurance, (4) % with Bachelor’s degree or higher degrees, (5) population density, (6) % Black residents, (7) % Hispanic residents, and (8) % with internet access.
One way to do this is to conduct a simple univariate comparison between the two groups. However, one would quickly realize that the high-income group has a fewer minority race than the low-income group, making the comparison biased. Many of the socioeconomic and racial variables are known to be correlated63,91,108. This means that univariate analysis of outcomes along one SDoH factor would likely be confounded by multiple other variables. In fact, within our dataset, we observed high correlation among many SDoH factors examined (Supplementary Table 3). For example, the median household income of the ZIP codes in our dataset is negatively correlated with the percentage of Black residents (Pearson r = − 0.23) and is positively correlated with internet access (Pearson r = 0.66). Comparing high and low-income groups without considering other factors would result in two groups of uneven distributions of race and internet access, among many other factors. Therefore, it is important to consider these factors jointly and adequately control for SES factors when analyzing outcome disparities63,91. To create a comparable and balanced set of groups with similar covariate distributions, we leverage matching-based methods.
Matching-based methods are commonly used to replicate randomized experiments as closely as possible in situations when randomized experiments are not possible from observational data109,110. This is achieved by obtaining balanced distribution of covariates in the treated and control groups109,111. Even though matching-based methods are commonly used for causal inferences, the same matching-based method can also be used to answer noncausal questions109 (e.g., racial disparities112). Our study, therefore, performs a longitudinal before-after observational study with matched groups to answer noncausal questions of the form: How did the changes in search behaviors during the pandemic differ across matched groups delineated by a single socioeconomic and environmental factor? In addition, our approach follows best practices for balancing comparison groups in longitudinal studies113 which we discuss in detail below.
In our study, we apply matching-based methods while considering the SDoH factors as treatments. Prior SDoH research suggests that the five SDoH are interrelated and impact one another114. Because of this relationship and known correlations between the SDoH factors, we consider all other SDoH factors as potential confounders of a selected treatment factor. It is true that considering SDoH factors as treatment poses challenges in the framing of the task because these factors are generally not modifiable (e.g., race) or difficult to modify (e.g., income). However, we refer to SDoH factors as treatments, not because they are modifiable, but because we apply the standard formulation of matching-based methods. Identifying modifiable factors in a matching-based experimental study can be used directly to make changes to those treatment factors and to reduce risk. On the other hand, identifying non-modifiable factors has been shown to also be useful to determine high-risk groups that require shielding and targeting for interventions87.
Because of the high degrees of spatial segregation in the US12,101, matching every ZIP code can be challenging. For example, for every ZIP code with low income and high proportions of Black residents, it is difficult to find a unique ZIP code with high-income and high proportions of Black residents. Therefore, we perform one-to-one matching of ZIP codes with replacement and achieve better matches (i.e., lower bias). Theoretically, this is at the expense of higher variance, but given the size of our dataset, this downside was not a problem in practice. We use the MatchIt package115 with the nearest neighbor method and Mahalanobis distance measure to perform the matching.
We leverage an extensive and iterative search across multiple matching methods to achieve maximum covariate balance and representative samples116. Regardless of which matching method is superior, one thing to note is that using a better matching method does not generally guarantee a better experimental design. It is then common practice to assess the quality of covariate balance, and in the end, it does not matter how this balance was achieved, as long as it was achieved. We choose to perform matching on all covariates, instead of propensity scoring117 which summarizes all of the covariates into one dimension. Importantly, we demonstrate in the Section Evaluating Quality of Matching Zip Codes that this method leads to high-quality matches that are balanced across all covariates.
Determining treatment and control groups
For each of the SDoH factors, we first split all available ZIP codes into treatment and control groups using a threshold. We use a value close to the median to split the population into two groups for median household income ($55,224), % unemployed (3.0%), % with insurance (92.7%), % with internet access (81.8%), and % with Bachelor’s degree or higher (21.1%) because the mean and median of those factors across the ZIP codes are similar. In other cases, the distribution across the ZIP codes is highly skewed. For race/ethnicity, we use the rounded percentage of the national population for that race/ethnicity (12% for Black and 18% for Hispanic residents). For population density, we follow previous practices of urban-rural classification at 500 people per square mile118. Supplementary Tables 1 and 2 outline descriptive statistics of our ZIP codes across SDoH factors as well as the national average and our chosen cutoff thresholds.
We consistently defined the treatment group as high-risk according to each of the dimensions of variation we specified68. Therefore, our treatment groups are as follows: low income, high percentage of minority residents, low level of educational attainment, high unemployment rate, low insurance rate, low level of internet access, and high population density. For example, for income, we split the ZIP codes into a high-income group (median household income>$55,224) and a low-income group (median household income ≤$55,224), where the low-income group is the treatment group. Then, for each treatment ZIP code, we look for a control (i.e., low-risk) ZIP code that closely matches it on all other SDoH factors (i.e., ∣SMD∣<0.25 to generate a matching pair of ZIP codes). We performed this matching on all ZIP codes, and we discarded ZIP codes for which we cannot find a good match. As demonstrated in Supplementary Table 6, this process retains at least 99.8% of the treatment ZIP codes in our matching process and the discarding of ZIP codes is a rare exception.
Evaluating quality of matching zip codes
To gauge whether two ZIP code groups are similar across the SDoH factors and to determine the quality of matching while minimizing the potential confounding effects of these factors, we leverage Standardized Mean Difference (SMD) across ZIP code groups as our measure of comparative quality. The SMD is used to quantify the degree to which two groups are different and is computed by the difference in means of a variable across two groups divided by the standard deviation of the one group (often, the treated group)111,119,120. In our analysis, we use ∣SMD∣ <0.25 across all our SDoH factors as a criterion to determine that the two groups are comparable, following common practice109,120. For example, when we split our ZIP codes in half along median household income to create a high-income ZIP code group (median household income >$55,224) and a low-income ZIP code group (median household income ≤$55,224) and examine the SMD of other SDoH factors, we find that all SDoH factors except % Hispanic residents and population density fail to achieve the necessary matching criteria of ∣SMD∣ < 0.25 prior to matching. This means that low-income ZIP codes are more likely to have less internet access, lower educational attainment level, less health insurance, more unemployment, and higher proportions of Black residents. We perform this evaluation process for all comparison groups to find that correlations among all SDoH factors pose threats to validity in univariate analyses. Supplementary Table 5 summarizes the mean SMD if we were to directly compare two ZIP code groups created by splitting the ZIP codes along the chosen split boundaries. Instead of such direct comparison, we perform matching and tune the caliper of the matching algorithm to determine a good match and to meet the ∣SMD∣<0.25 criterion between the two comparison groups across all covariates. Supplementary Table 6 summarizes the result of the matching operation with the maximum ∣SMD∣ being below 0.25, that is ensuring comparability across all covariates, between two ZIP code groups along all SDoH factors. Supplementary Tables 7–22 enumerate pre- and post-matching balance assessments between groups for each SDoH factor.
Estimating the effect size
After identifying treatment and control ZIP code groups with comparable distributions along all SDoH factors, we compare the outcomes (i.e., constructs of digital engagement such as online access to health condition information) between the matched ZIP code groups. This matching process estimates, for example, the differences in the changes in online health information-seeking behaviors between high and low-income groups during the pandemic while removing plausible contributions from all other observed factors. The differences estimated in this study help identify high-risk groups (e.g., low income, low educational attainment, high proportions of minority residents) for whom to suggest interventions or targeted shielding mitigate or reduce risk87.
It is important to note that our matching process only partially incorporates what Helsper calls the digital impact mediators of access, skills, and attitudes57. First, where digital access is concerned, though all search queries in the study presume some form of internet access, we do sample ZIP codes with varying levels of aggregate internet access, allowing us to control to some extent for internet access at the population level. It is important to note, however, that our study lacks the data to account for any changes in ZIP code-level internet access during the pandemic due to remote work. Where digital skills are concerned, we do not incorporate direct measures of such technical or operational skills at either the individual or aggregate level, but we do incorporate measures of educational attainment such that we can partially control for this factor in our analysis. Finally, we do not control for individual-level or aggregate-level variation in attitudinal impact mediators such as self-efficacy, as that would be outside the scope of the study. Additional more detailed data would have to be collected and analyzed in order to fully disentangle the impacts of the SDoH factors under study here from such digital impact mediators.
Raw data were collected by proprietary code through Microsoft Bing platform. Study data were extracted from Bing search logs stored on Microsoft’s internal database and processed using its proprietary query language. Data analysis was conducted in Python (v3.9.6) using standard data analysis libraries such as numpy (v1.20.3), scipy (v1.6.2), and pandas (v1.3.1). Visualization was produced using seaborn (v0.11.1). Matching was done using MatchIt (v4.2.0) in R.
Reporting summary
Further information on research design is available in the Nature Portfolio Reporting Summary linked to this article.